In the realm of financial markets, where uncertainty and volatility reign supreme, quantitative options trading emerges as a beacon of precision and profitability. It’s a data-driven investment strategy that harnesses the power of mathematics and computer algorithms to navigate the complexities of options markets, enhancing decision-making and unlocking exceptional returns. In this comprehensive guide, we delve into the fascinating world of quantitative options trading, exploring its history, foundational concepts, and practical applications.
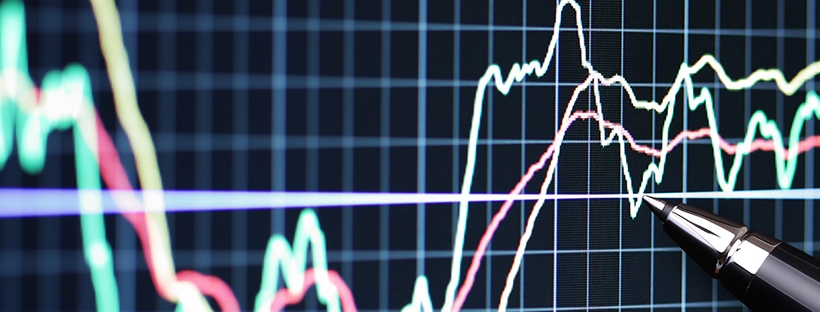
Image: mwambaanalytics.com
Understanding Quantitative Options Trading
Quantitative options trading is a sophisticated strategy that utilizes sophisticated mathematical models and statistical techniques to analyze vast amounts of market data. Unlike traditional options trading, which often relies on subjective judgment and intuition, quantitative options trading employs algorithmically driven models to identify patterns, forecast market movements, and optimize trading strategies. This data-centric approach allows traders to make informed decisions based on objective analysis, reducing the element of human bias and enhancing risk management.
Historical Roots in Academia and Industry
The roots of quantitative options trading can be traced back to the academic community. In the 1970s, renowned mathematicians, such as Fischer Black and Myron Scholes, developed groundbreaking models, including the Black-Scholes model, which revolutionized the pricing of options. These models became the cornerstone of modern options theory and served as the foundation for quantitative options trading.
As the financial industry embraced the power of computing, quantitative options trading gained momentum in the 1980s and 1990s. Wall Street firms invested heavily in developing proprietary trading systems and hiring quants, mathematicians, and physicists to build sophisticated trading models. The rise of electronic trading platforms in the early 2000s further accelerated the adoption of quantitative options trading, enabling traders to execute complex strategies with greater speed and efficiency.
Core Concepts of Quantitative Options Trading
At the heart of quantitative options trading lies a deep understanding of options pricing models and statistical techniques. Options contracts, which give the holder the right but not the obligation to buy or sell an underlying asset at a specified price on a specified date, are inherently complex and require advanced mathematical tools to value accurately.
Quantitative options traders employ a diverse range of modeling techniques, including Monte Carlo simulations, binomial trees, and finite difference methods, to price options and forecast future market movements. They also utilize statistical analysis, such as time series analysis and regression modeling, to identify patterns in historical data and make predictions about future market behavior.

Image: www.goodreads.com
Diverse Trading Strategies Based on Underlying Factors
Quantitative options traders employ a wide spectrum of trading strategies, each tailored to capture specific market inefficiencies and profit from anticipated market movements. Some of the most common strategies include:
- **Delta-neutral strategies:** Aiming to eliminate exposure to price movements of the underlying asset while profiting from changes in implied volatility.
- **Theta-based strategies:** Exploiting the time decay of options premia to generate returns.
- **Volatility arbitrage strategies:** Capitalizing on discrepancies between the implied and realized volatility of the underlying asset.
- **Event-driven strategies:** Leveraging anticipated events, such as earnings announcements or mergers, to trade options on companies expected to be affected by the events.
Technological Advancements Fueling Model Complexity
The rapid pace of technological advancements in recent years has fueled significant strides in the development of quantitative options trading models. The availability of vast amounts of historical market data and the emergence of powerful computing resources has enabled the creation of highly complex and sophisticated models that can handle the immense computational demands of modern options trading.
Machine learning (ML) and artificial intelligence (AI) techniques have further enhanced the capabilities of quantitative options trading models. ML models can learn from historical data to identify patterns and make predictions that are not easily captured by traditional models. AI algorithms can automate the process of model development and optimization, allowing traders to explore a wider range of strategies and adapt to changing market conditions with greater agility.
Risk Management in Quantitative Options Trading
Risk management is of paramount importance in quantitative options trading, given the inherent volatility and potential for significant losses. Quantitative options traders employ various risk management techniques to control and mitigate risks, including:
- **Stress testing:** Simulating extreme market conditions to assess the resilience of trading strategies.
- **Value-at-Risk (VaR) analysis:** Quantifying the potential loss in a trading portfolio under specified confidence levels.
- **Position sizing:** Determining the optimal size of trades based on risk tolerance and portfolio diversification.
- **Diversification:** Spreading investments across a range of assets and strategies to reduce risk.
Quantitative Options Trading
Conclusion: Unlocking Exceptional Returns through Data-Driven Investment
Quantitative options trading has revolutionized the world of options trading by introducing a data-driven and scientific approach to investment decision-making. By harnessing the power of mathematics, computer algorithms, and vast amounts of market data, quantitative options traders have gained a significant edge in navigating the complexities of options markets. This sophisticated trading strategy offers the potential for exceptional returns while enabling traders to manage risk more effectively. As technology continues to advance and the availability of data